정보
-
업무명 : 2019년 주요 도시의 월별 오존 농도 데이터를 이용하여 계절별 오존 농도 차이 여부 검정
-
작성자 : 박진만
-
작성일 : 2021-02-25
-
설 명 :
-
수정이력 :
내용
[개요]
-
안녕하세요? 기상 연구 및 웹 개발을 담당하고 있는 해솔입니다.
-
이번 포스팅에서는 2019년 각 주요도시의 월별 오존 농도 데이터를 이용하여 통계적 분석을 수행 하도록 하겠습니다.
-
상기 데이터를 도시별/계절별로 나눈 후 계절별 오존 농도의 차이가 존재하는지 확인하고 이를 시계열 및 상자그림의 형태로 나타내었습니다.
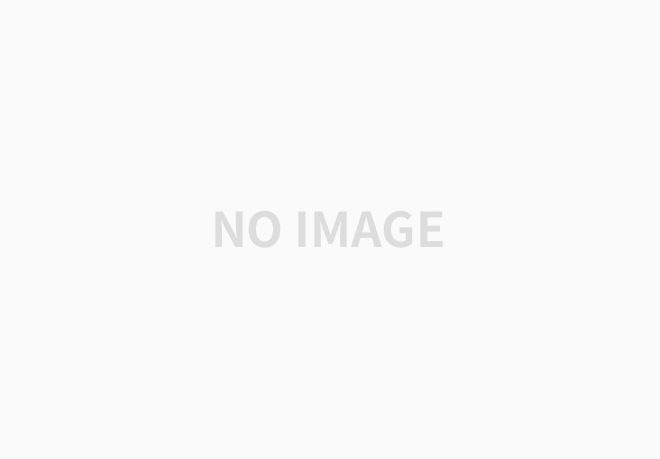
[특징]
-
R을 이용한 데이터 전처리 및 통계적 분석을 수행하고 이를 시각화 한다.
[기능]
-
데이터 로드 및 전처리
-
정규성 검정 및 wilcoxon 검정
-
ggplot을 통한 시각화 (시계열 및 상자그림)
[활용 자료]
-
2019년 주요도시 오존 데이터
[자료 처리 방안 및 활용 분석 기법]
-
없음
[사용법]
-
소스 코드 참조
[사용 OS]
-
Windows 10
[사용 언어]
-
R v4.0.3
소스 코드
[명세]
- 라이브러리 로드
library(tidyverse)
library(openxlsx)
library(lubridate)
library(region5air)
library(openair)
library(TTR)
library(ggplot2)
- 데이터 로드
-
월별 오존 농도 자료 읽기
-
데이터의 구조는 아래의 이미지와 같음
-
오존의 농도는 ppm 단위임
-
# 데이터 로드
data = openxlsx::read.xlsx("./INPUT/오존오염도최종.xlsx", sheet = 2)
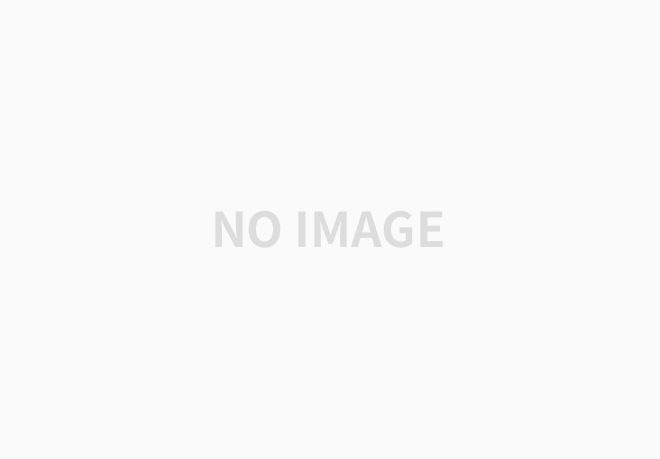
-
전체 데이터 전처리
-
전체 지점에 대한 월별 시계열을 그리기 위하여 tidyr 패키지의 gather 함수를 이용하여 데이터를 모아주는 형태로 가공
-
가공이 끝난 데이터는 key와 value 를 중심으로 하나로 모인 형태가 됨
-
# 전체 지역에 대한 월별 시계열 시각화
dataL1 = data %>%
tidyr::gather(-연,-월, key = "key", value = "value")
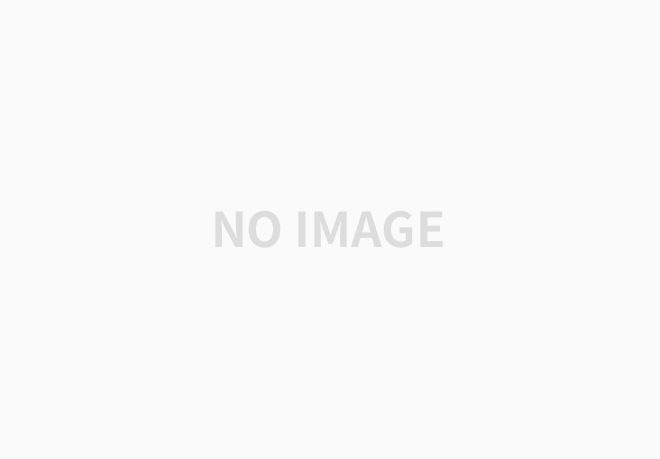
-
1월 ~ 12월 까지의 주요 도시 오존 농도 시계열 시각화
-
ggplot 을 이용하여 주요 도시에 대한 월별 시계열 생성
-
# 전체 도시에 대한 시계열 그리기
ggplot(dataL1, aes(`월`, value, group=key, colour=key)) +
theme_bw() +
theme(plot.title=element_text(face="bold", size=20, color="black")) +
theme(axis.title.x = element_text(face="bold", size=15, colour="black")) +
theme(axis.title.y = element_text(face="bold", size=15, colour="black", angle=90)) +
theme(axis.text.x = element_text(face="bold", size=14, colour="black")) +
theme(axis.text.y = element_text(face="bold", size=14, colour="black")) +
theme(legend.title=element_text(face="bold", size=15, colour="black")) +
theme(legend.key=element_blank()) +
theme(legend.text=element_text(size=15, face="bold")) +
theme(legend.background=element_blank()) +
geom_line(size = 1) +
geom_point(size = 2) +
scale_x_continuous(breaks = seq(1,12,1),limits = c(1,12)) +
labs(title = "월별 오존농도 (도시별)",
x = "월 (month)",
y = "오존 농도 (ppm)") +
ggsave("./월별 오존농도.png",dpi = 600)
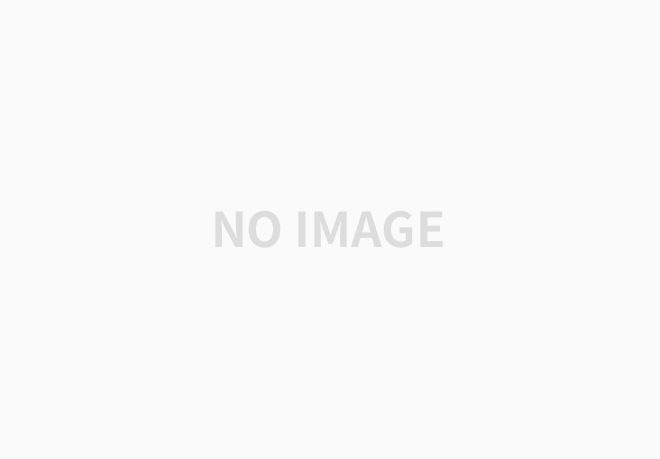
-
계절별로 오존 데이터 분할 후 상자그림 그려보기
-
패키지는 ggplot 패키지를 그대로 사용
-
봄 (3,4,5월), 여름 (6,7,8월), 가을(9,10,11월), 겨울(12,1,2월) 로 데이터를 분할 후 상자그림을 그림
-
이 과정에서 총합에 해당하는 데이터는 제거 하였음
-
# 겨울 데이터 추출 (12,1,2)
dataL2_winter = dataL1 %>%
dplyr::filter(key != "총계") %>%
dplyr::filter(`월` == 12 | `월` == 1 | `월` == 2)
# 봄 데이터 추출 (3,4,5)
dataL2_spring = dataL1 %>%
dplyr::filter(key != "총계") %>%
dplyr::filter(`월` == 3 | `월` == 4 | `월` == 5)
# 여름 데이터 추출 (6,7,8)
dataL2_summer = dataL1 %>%
dplyr::filter(key != "총계") %>%
dplyr::filter(`월` == 6 | `월` == 7 | `월` == 8)
# 가을 데이터 추출 (9,10,11)
dataL2_fall = dataL1 %>%
dplyr::filter(key != "총계") %>%
dplyr::filter(`월` == 9 | `월` == 10 | `월` == 11)
## box plot ##
ggplot() +
theme_bw() +
theme(plot.title=element_text(face="bold", size=20, color="black")) +
theme(axis.title.x = element_text(face="bold", size=15, colour="black")) +
theme(axis.title.y = element_text(face="bold", size=15, colour="black", angle=90)) +
theme(axis.text.x = element_text(face="bold", size=14, colour="black")) +
theme(axis.text.y = element_text(face="bold", size=14, colour="black")) +
theme(legend.title=element_text(face="bold", size=15, colour="black")) +
theme(legend.key=element_blank()) +
theme(legend.text=element_text(size=15, face="bold")) +
theme(legend.background=element_blank()) +
geom_boxplot(data = dataL2_spring,aes(x = 1,y = value)) +
geom_boxplot(data = dataL2_summer,aes(x = 2,y = value)) +
geom_boxplot(data = dataL2_fall,aes(x = 3,y = value)) +
geom_boxplot(data = dataL2_winter,aes(x = 4,y = value)) +
scale_x_continuous(breaks = seq(1,4,1),labels = c("봄","여름","가을","겨울")) +
labs(title = "계절에 따른 도시 오존농도 분포",
x = "계절",
y = "오존 농도 (ppm)") +
ggsave("./계절별 오존 농도 분포.png",dpi = 600)
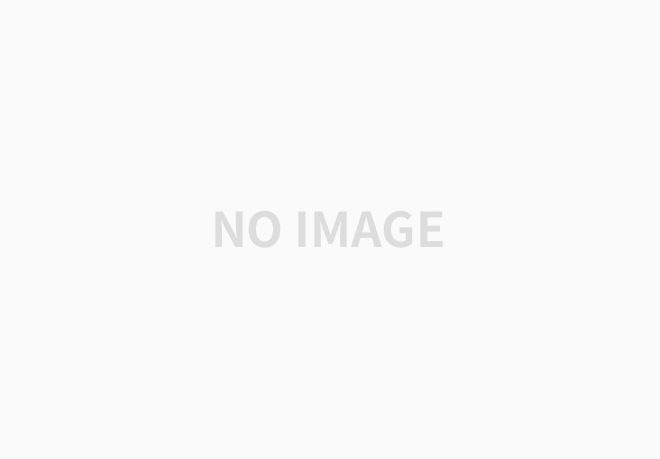
-
각 월별 데이터의 정규성 검정
-
kolmogorov-Smirnov test (콜모고로프-스미노프 검정) 수행
-
자료의 평균/표준편차와 히스토그램을 표준정규분포와 비교하여 적합도를 검정하는 방법
-
결과적으로 p-value가 0.05보다 큰 경우 정규성이 있다고 가정할 수 있음
-
위와 같은 검정을 수행하는 이유는 데이터가 정규성을 만족하는 경우 anova 검정 수행이 가능하지만 그렇지 않는 경우 다른 검정 방법이 필요하기 때문임
-
검정 결과 오존 데이터의 경우 정규성을 띠지는 않는 것으로 확인
-
이는 계절별 차이를 데이터의 비정규성을 가정하는 wilcoxon 검정을 수행 해야 함을 의미함
-
-
## 각 데이터의 정규성 검정 ##
# 계절별 검정 수행
x = dataL2_spring$value
ks.test(x ,"pnorm",mean = mean(x),sd = sd(x))
# One-sample Kolmogorov-Smirnov test
#
# data: x
# D = 0.12967, p-value = 0.3578
# alternative hypothesis: two-sided
x = dataL2_summer$value
ks.test(x ,"pnorm",mean = mean(x),sd = sd(x))
# One-sample Kolmogorov-Smirnov test
#
# data: x
# D = 0.17878, p-value = 0.07675
# alternative hypothesis: two-sided
x = dataL2_fall$value
ks.test(x ,"pnorm",mean = mean(x),sd = sd(x))
# data: x
# D = 0.14623, p-value = 0.2255
# alternative hypothesis: two-sided
x = dataL2_winter$value
ks.test(x ,"pnorm",mean = mean(x),sd = sd(x))
# data: x
# D = 0.11338, p-value = 0.5285
# alternative hypothesis: two-sided
-
Wilcoxon 검정
-
각 계절별로 분할된 데이터를 이요하여 Wilcoxon 검정을 통해 데이터간 평균에 차이가 있는지 검정
-
즉 가설 지정 : 계절이 오존 농도의 변화에 영향을 미치는가? 이며
-
귀무가설 : 계절에 따른 오존 농도의 차이가 없다.
-
대립가설 : 계절에 따른 오존 농도의 차이가 있다. 로 요약 가능
-
-
검정 코드 및 결과는 아래와 같으며 결과는 p-value 가 0.05보다 작으므로 유의수준에서 귀무가설이 기각됨
-
즉 계절에 따른 오존 농도의 차이가 있다는 결론을 얻음
-
# 분석을 위한 데이터 병합 및 그룹 지정
dataL2_spring$season = "봄"
dataL2_summer$season = "여름"
dataL2_fall$season = "가을"
dataL2_winter$season = "겨울"
data_L3 = rbind(dataL2_spring,dataL2_summer,dataL2_fall,dataL2_winter)
## 두 계절씩 나누어 반복테스트 수행
seasonis = c("봄","여름","가을","겨울")
seasoniscombin = combn(seasonis,2)
result = data.frame()
for (i in 1:dim(seasoniscombin)[2]) {
data_L4 = data_L3 %>%
dplyr::filter(season == seasoniscombin[1,i] | season == seasoniscombin[2,i])
test <- wilcox.test(data_L4$value ~ data_L4$season)
result_part = data.frame(season1 = seasoniscombin[1,i], season2 = seasoniscombin[2,i], pval = test$p.value)
result = rbind(result,result_part)
}
print(result)
## 결과 ##
# season1 season2 pval
# 1 봄 여름 8.836458e-05
# 2 봄 가을 1.526048e-15
# 3 봄 겨울 6.450829e-16
# 4 여름 가을 2.429450e-14
# 5 여름 겨울 4.675901e-14
# 6 가을 겨울 7.858701e-03
[전체 코드]
#===============================================================================================
options(warn = -1)
library(tidyverse)
library(openxlsx)
library(lubridate)
library(region5air)
library(openair)
library(TTR)
library(ggplot2)
# 데이터 로드
data = openxlsx::read.xlsx("./INPUT/오존오염도최종.xlsx", sheet = 2)
# 전체 지역에 대한 월별 시계열 시각화
dataL1 = data %>%
tidyr::gather(-연,-월, key = "key", value = "value")
# 전체 도시에 대한 시계열 그리기
ggplot(dataL1, aes(`월`, value, group=key, colour=key)) +
theme_bw() +
theme(plot.title=element_text(face="bold", size=20, color="black")) +
theme(axis.title.x = element_text(face="bold", size=15, colour="black")) +
theme(axis.title.y = element_text(face="bold", size=15, colour="black", angle=90)) +
theme(axis.text.x = element_text(face="bold", size=14, colour="black")) +
theme(axis.text.y = element_text(face="bold", size=14, colour="black")) +
theme(legend.title=element_text(face="bold", size=15, colour="black")) +
theme(legend.key=element_blank()) +
theme(legend.text=element_text(size=15, face="bold")) +
theme(legend.background=element_blank()) +
geom_line(size = 1) +
geom_point(size = 2) +
scale_x_continuous(breaks = seq(1,12,1),limits = c(1,12)) +
labs(title = "월별 오존농도 (도시별)",
x = "월 (month)",
y = "오존 농도 (ppm)") +
ggsave("./월별 오존농도.png",dpi = 600)
#===============================================================================================
# 주기가 1년 (즉 한 개의 주기) 으로 보여지기 때문에 시계열로부터 구체적인 주기성을
# 추출 할 수는 없음 -> 전체 도시로부터 계절별로 나눈 후 아노바 검정을 수행해 분산분석을 하는 방법 사용
# 겨울 (12,1,2) 월
# 봄 (3,4,5) 월
# 여름 (6,7,8) 월
# 가을 (9,10,11) 월 롤 데이터를 나눈 후 자료의 특성이 다른지 확인 하고자 함
## 총합은 제외함 ##
# 겨울 데이터 추출 (12,1,2)
dataL2_winter = dataL1 %>%
dplyr::filter(key != "총계") %>%
dplyr::filter(`월` == 12 | `월` == 1 | `월` == 2)
# 봄 데이터 추출 (3,4,5)
dataL2_spring = dataL1 %>%
dplyr::filter(key != "총계") %>%
dplyr::filter(`월` == 3 | `월` == 4 | `월` == 5)
# 여름 데이터 추출 (6,7,8)
dataL2_summer = dataL1 %>%
dplyr::filter(key != "총계") %>%
dplyr::filter(`월` == 6 | `월` == 7 | `월` == 8)
# 가을 데이터 추출 (9,10,11)
dataL2_fall = dataL1 %>%
dplyr::filter(key != "총계") %>%
dplyr::filter(`월` == 9 | `월` == 10 | `월` == 11)
## box plot ##
ggplot() +
theme_bw() +
theme(plot.title=element_text(face="bold", size=20, color="black")) +
theme(axis.title.x = element_text(face="bold", size=15, colour="black")) +
theme(axis.title.y = element_text(face="bold", size=15, colour="black", angle=90)) +
theme(axis.text.x = element_text(face="bold", size=14, colour="black")) +
theme(axis.text.y = element_text(face="bold", size=14, colour="black")) +
theme(legend.title=element_text(face="bold", size=15, colour="black")) +
theme(legend.key=element_blank()) +
theme(legend.text=element_text(size=15, face="bold")) +
theme(legend.background=element_blank()) +
geom_boxplot(data = dataL2_spring,aes(x = 1,y = value)) +
geom_boxplot(data = dataL2_summer,aes(x = 2,y = value)) +
geom_boxplot(data = dataL2_fall,aes(x = 3,y = value)) +
geom_boxplot(data = dataL2_winter,aes(x = 4,y = value)) +
scale_x_continuous(breaks = seq(1,4,1),labels = c("봄","여름","가을","겨울")) +
labs(title = "계절에 따른 도시 오존농도 분포",
x = "계절",
y = "오존 농도 (ppm)") +
ggsave("./계절별 오존 농도 분포.png",dpi = 600)
#===============================================================================================
## 각 데이터의 정규성 검정 ##
# Kolmogorov-Smirnov test, 콜모고로프-스미노프 검정 수행
# 자료의 평균/표준편차와 히스토그램을 표준정규분포와 비교하여 적합도를 검정하는 방법이며
# p-value가 0.05보다 크면 정규성을 가정하게 된다.
# 계절별 검정 수행
x = dataL2_spring$value
ks.test(x ,"pnorm",mean = mean(x),sd = sd(x))
# One-sample Kolmogorov-Smirnov test
#
# data: x
# D = 0.12967, p-value = 0.3578
# alternative hypothesis: two-sided
x = dataL2_summer$value
ks.test(x ,"pnorm",mean = mean(x),sd = sd(x))
# One-sample Kolmogorov-Smirnov test
#
# data: x
# D = 0.17878, p-value = 0.07675
# alternative hypothesis: two-sided
x = dataL2_fall$value
ks.test(x ,"pnorm",mean = mean(x),sd = sd(x))
# data: x
# D = 0.14623, p-value = 0.2255
# alternative hypothesis: two-sided
x = dataL2_winter$value
ks.test(x ,"pnorm",mean = mean(x),sd = sd(x))
# data: x
# D = 0.11338, p-value = 0.5285
# alternative hypothesis: two-sided
## 결과적으로 모두 정규성을 띠지 않는 것으로 확인됨 ##
#===============================================================================================
# 따라서 Wilcoxon검정을 통해 비정규성을 가정해 그룹간 차이를 비교하는 방법을 사용
# 가설 지정 : 계절이 오존 농도에 영향을 미치는가?
# 귀무가설 : 계절에 따른 오존 농도의 차이가 없다.
# 대립가설 : 계절에 따른 오존 농도의 차이가 있다.
# 분석을 위한 데이터 병합 및 그룹 지정
dataL2_spring$season = "봄"
dataL2_summer$season = "여름"
dataL2_fall$season = "가을"
dataL2_winter$season = "겨울"
data_L3 = rbind(dataL2_spring,dataL2_summer,dataL2_fall,dataL2_winter)
## 두 계절씩 나누어 반복테스트 수행
seasonis = c("봄","여름","가을","겨울")
seasoniscombin = combn(seasonis,2)
result = data.frame()
for (i in 1:dim(seasoniscombin)[2]) {
data_L4 = data_L3 %>%
dplyr::filter(season == seasoniscombin[1,i] | season == seasoniscombin[2,i])
test <- wilcox.test(data_L4$value ~ data_L4$season)
result_part = data.frame(season1 = seasoniscombin[1,i], season2 = seasoniscombin[2,i], pval = test$p.value)
result = rbind(result,result_part)
}
print(result)
참고 문헌
[논문]
- 없음
[보고서]
- 없음
[URL]
- 없음
문의사항
[기상학/프로그래밍 언어]
- sangho.lee.1990@gmail.com
[해양학/천문학/빅데이터]
- saimang0804@gmail.com
'프로그래밍 언어 > R' 카테고리의 다른 글
[R] RSelenium을 이용한 네이버 쇼핑몰 댓글 스크래핑 (0) | 2021.05.18 |
---|---|
[R] R을 이용하여 국가기상위성센터의 위성영상 자료 다운로드 하기 (1) | 2021.02.06 |
[R] R 및 flourish studio 를 이용하여 국가별 탄소 배출량 레이싱 차트 만들기 (0) | 2021.02.01 |
[R] 격자정보 및 U,V,풍속 정보를 이용하여 동아시아 지도에 매핑하기 (0) | 2021.01.30 |
[R] WRF 모델자료 후처리 시퀀스1 - nc 파일을 풀어 txt 형태로 떨어뜨리기 (0) | 2021.01.27 |